

Specifically, a novel pyramid structure loss is proposed to supervise structure learning and embedding. In the meantime, we also introduce a structure embedding scheme to explicitly embed the learned structure features into the inpainting process, thus to provide possible preconditions for image completion.
Image inpaint generator#
The primary idea is to train a shared generator to simultaneously complete the corrupted image and corresponding structures - edge and gradient, thus implicitly encouraging the generator to exploit relevant structure knowledge while inpainting.

This paper develops a multi-task learning framework that attempts to incorporate the image structure knowledge to assist image inpainting, which is not well explored in previous works.
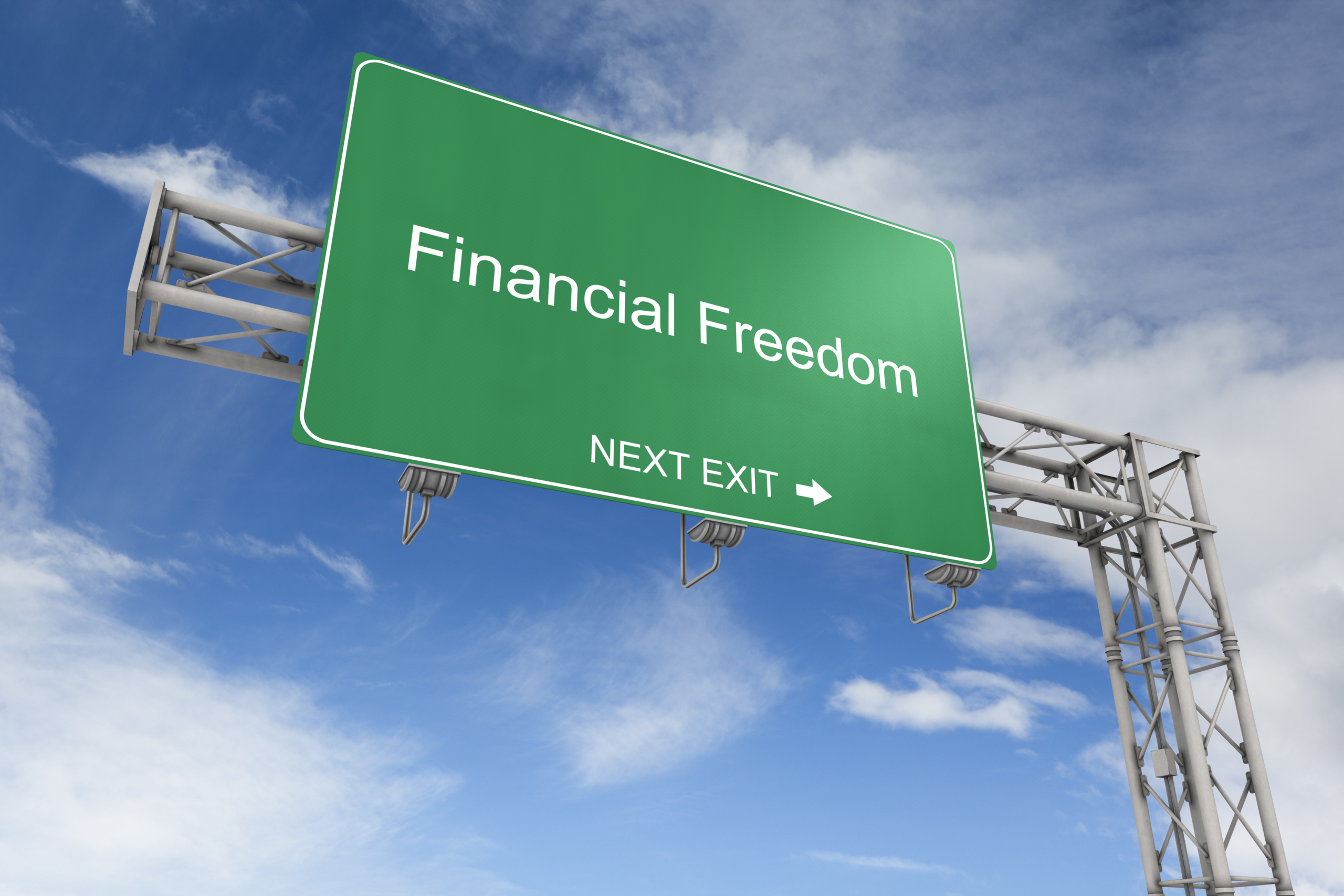
The overall quality of the edited image is not inferior to the other face attribute editing method, and attribute classification for edited image shows a 92.6% attribute editing success rate of the proposed method. These experiments prove that our method can edit a variety of face images that vary with race, gender, age, and camera shooting angle. Images of the FFHQ dataset are edited by attribute labels defined in Celeba dataset for experiments. Secondly, by editing the latent variable of the GAN, the attribute of the generated face image can be modified indirectly, so it is almost unaffected by the training process and network structure of GAN, which means it is a flexible method for nearly all GAN network. In particular, face attribute editing is divided into two separate stages: Firstly, based on the optimization function, the generative model does a latent variable search to generate a high-quality face image that is similar to the input image. In this paper, a method to edit face attributes by editing the latent variable with the help of a pre-trained unconditional GAN and a linear classification model is proposed. However, the existing methods cannot control the editing content of the face elements according to the user-specified attributes or need to train a conditional GAN for editing tasks, which means it is difficult to add new attributes in the future. Recently, a variety of methods using the Generative Adversarial Network (GAN) for face editing have been proposed. Applications of this technique include the restoration of old photographs and damaged film removal of superimposed text like dates, subtitles, or publicity and the removal of entire objects from the image like microphones or wires in special effects. In addition, no limitations are imposed on the topology of the region to be inpainted. This is automatically done (and in a fast way), thereby allowing to simultaneously fill-in numerous regions containing completely different structures and surrounding backgrounds. In contrast with previous approaches, the technique here introduced does not require the user to specify where the novel information comes from. The fill-in is done in such a way that isophote lines arriving at the regions' boundaries are completed inside.

After the user selects the regions to be restored, the algorithm automatically fills-in these regions with information surrounding them.
Image inpaint professional#
In this paper, we introduce a novel algorithm for digital inpainting of still images that attempts to replicate the basic techniques used by professional restorators. The goals and applications of inpainting are numerous, from the restoration of damaged paintings and photographs to the removal/replacement of selected objects. Inpainting, the technique of modifying an image in an undetectable form, is as ancient as art itself.
